A Trick to Remember
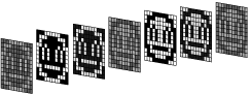
The human brain has been called the most complex object in the Universe, so it’s no surprise that researchers have yet to understand how it stores memories. Yet physicists hope to learn something about memory by studying simplified computer models called neural networks, which have some properties in common with real brains. In the 12 March PRL researchers correct a serious flaw in a type of neural network that aims to better simulate real neuron behavior, and show that it can retrieve stable “memories.” Networks such as these demonstrate that complex, brain-like behavior can arise from a collection of simple components, and they remain the best computer model for the operation of memory.
In the early 1980s, John Hopfield, now at Princeton University, proposed a type of neural network with a capacity for recalling patterns, or “memories.” It consists of “neurons” that are assigned values of one or minus one, based on a weighted average of the values of all the other neurons. If you start by assigning values to each neuron and then let the system go on its own, the values change with time as the neurons continually respond to one another. But eventually the network settles down to an unchanging pattern of values. Each choice of weightings creates a network with several stable “template” patterns–dubbed “memories”–of neuron values. When you start the network in some other pattern, it moves to the closest template pattern–it “recalls” the closest memory.
A real neuron “at rest,” however, doesn’t maintain a fixed voltage value; it fires repeated voltage spikes at a fixed rate. So researchers have devised networks whose elements are coupled entities whose values oscillate in time. For example, a memory state might consist of neurons oscillating in phase or exactly out of phase, rather than remaining as a static pattern.
But these more realistic networks suffer from a stability problem, explains Takashi Nishikawa of Southern Methodist University in Dallas. The desired memory states are no more stable than other states that the network can fall into. When presented with some new pattern, the oscillatory network, unlike the original Hopfield model, does not automatically move toward the memory state closest to that pattern.
Nishikawa and his colleagues at Arizona State University in Tempe have found a simple way to correct this deficiency. They changed the weighting formula–which depends on phase differences between neurons–to give more weight to neurons when they are at certain phases of their cycles. Increasing the magnitude of this extra weight makes the memory patterns of the network stable, the researchers found. However, if the extra weight is too strong, other, random states of the network also become stable. When that happens, the network may not produce a memory state in response to an input pattern. But when the extra weight is adjusted correctly, the researchers concluded, the network shows a recall ability comparable to the classical Hopfield network.
How any of this relates to real neurological memory is hard to know, Nishikawa admits. Synchronous firing of biological neurons is only one possible way to encode memories, and the connections between neurons that underlie such phenomena are poorly understood. Zhaoping Li of University College London suggests that the techniques devised by Nishikawa’s team may in the end have more value for computer science and engineering than for neuroscience. Nevertheless, she adds, it will take ideas from all these disciplines to bring about a genuine understanding of memory and other brain functions.
–David Lindley
David Lindley is a freelance science writer, now retired. His most recent book is The Dream Universe: How Fundamental Physics Lost Its Way (Penguin Random House, 2020).