Vaccinate Thy Neighbor
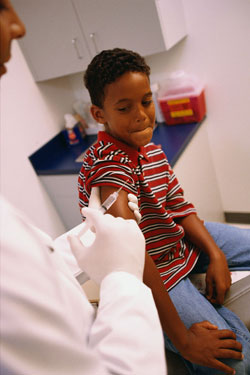
When vaccine supplies for a deadly disease are limited, whom should health workers target? In the 12 December PRL, researchers describe a vaccination strategy that finds the individuals most likely to spread the disease, without having any advanced knowledge of who they are. The strategy could be applied to computer viruses as easily as human ones and may also suggest a strategy for breaking up terrorist networks.
Human networks of acquaintances, computer networks like the Internet, and interacting protein networks in the body, all share a characteristic layout: most of the elements have only a few links to others, while a few individuals have a very large number of links. If one of these highly connected individuals in a human network becomes infected, she can become a “super-spreader,” infecting all of her contacts and efficiently distributing the disease. This structure suggests a deceptively simple solution to the vaccination question: immunizing all the super-spreaders in a network slows or stops the spread of a disease as effectively as destroying a country’s highway interchanges would stop traffic.
Unfortunately, it’s rarely obvious which individuals are well-connected. People rarely know exactly how many contacts they have and sometimes lie, particularly about sensitive subjects like sexual partners. Random immunization is almost useless because, for many viruses, more than 95% of the population must be vaccinated to prevent the disease’s spread.
Reuven Cohen of Bar-Ilan University in Israel and his colleagues propose a simple modification of random vaccination that is more effective, according to their computer simulations. The idea is to randomly choose, say, 20% of the individuals and ask them to name one acquaintance; then vaccinate those acquaintances. Potential super-spreaders have such a large number of acquaintances that they are very likely to be named at least once, the researchers found. On the other hand, the super-spreaders are so few in number that the random 20% of individuals is unlikely to include many of them.
Using the team’s vaccination strategy, a disease can be stopped by vaccinating less than 20% of the individuals, in some cases, according to their computer model of a human population. The method can also be tweaked: if a larger sample is asked for names, and those named twice are vaccinated, the total number of vaccinations required can be even lower.
The work could have “enormous practical potential” in regions such as South Africa, where there are not enough drugs to treat all who are at risk, says physicist Albert-Laszlo Barabasi, who studies disease and other networks at the University of Notre Dame in Indiana. Cohen says the strategy could be adapted to solve problems beyond immunization, including making computer networks more stable and web searches more efficient. His group also suggests a strategy for efficiently breaking up terrorist networks by focusing on removing acquaintances named by known terrorists.
–Kim Krieger
Kim Krieger is a freelance science writer in Norwalk, Connecticut.